概述
中介中心性(Betweenness Centrality)量化一个点位于图中其它两点间最短路径上的概率。这个指标能有效地找到在图的各部分间起桥梁作用的点。
中介中心性的取值范围是0到1,分值越大,点对于网络流通性或连通性的影响力越大。
参考资料:
- L.C. Freeman, A Set of Measures of Centrality Based on Betweenness (1977)
- L.C. Freeman, Centrality in Social Networks Conceptual Clarification (1978)
基本概念
最短路径
两点间的最短路径是指它们之间边数最少的路径。中介中心性算法的最短路径不考虑边权重。
中介中心性
点x
的中介中心性计算公式为:
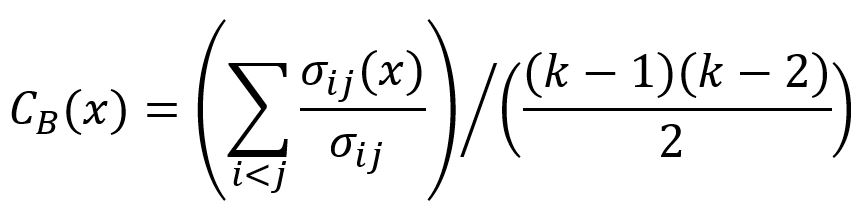
其中,
i
和j
是图中互异的两个点,不包括x
。σij
为i
、j
间最短路径的数量。σij(x)
为i
、j
间经过x
的最短路径的数量。σij(x)/σij
就是x
位于i
、j
间最短路径的概率。注意,i
、j
不连通时,σij(x)/σij
为0。k
是图中点的总数,ij
点对的数量就是(k-1)(k-2)/2
。
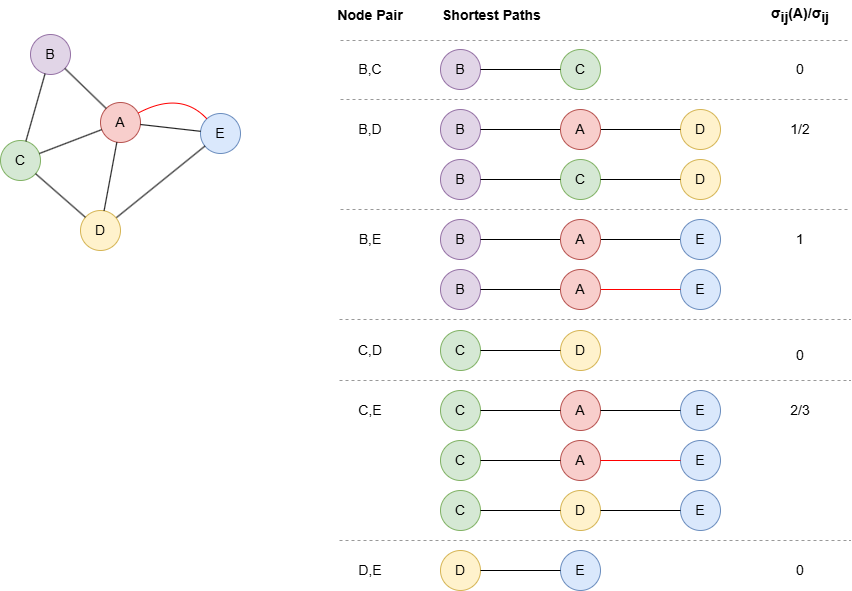
点A
的中介中心性为:(0 + 1/2 + 1 + 0 + 2/3 + 0) / 6 = 0.3611
。
采样计算
在规模较大的图上运行本算法会消耗很多计算资源。当图中点数量超过一万时,建议按点或边采样后进行近似计算。算法只进行一次均匀采样。
特殊说明
- 孤点的中介中心性为0。
- 中介中心性算法忽略边方向。未进行采样时,在包含
k
个点的图中,参与计算的点对数量是(k-1)(k-2)/2
。
示例图集
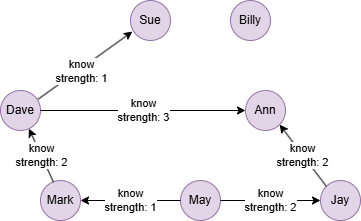
创建示例图集:
// 在空图集中逐行运行以下语句
create().node_schema("user").edge_schema("know")
insert().into(@user).nodes([{_id:"Sue"}, {_id:"Dave"}, {_id:"Ann"}, {_id:"Mark"}, {_id:"May"}, {_id:"Jay"}, {_id:"Billy"}])
insert().into(@know).edges([{_from:"Dave", _to:"Sue"}, {_from:"Dave", _to:"Ann"}, {_from:"Mark", _to:"Dave"}, {_from:"May", _to:"Mark"}, {_from:"May", _to:"Jay"}, {_from:"Jay", _to:"Ann"}])
在HDC图集上运行算法
创建HDC图集
将当前图集全部加载到HDC服务器hdc-server-1
上,并命名为 hdc_betweenness
:
CALL hdc.graph.create("hdc-server-1", "hdc_betweenness", {
nodes: {"*": ["*"]},
edges: {"*": ["*"]},
direction: "undirected",
load_id: true,
update: "static",
query: "query",
default: false
})
hdc.graph.create("hdc_betweenness", {
nodes: {"*": ["*"]},
edges: {"*": ["*"]},
direction: "undirected",
load_id: true,
update: "static",
query: "query",
default: false
}).to("hdc-server-1")
参数
算法名:betweenness_centrality
参数名 |
类型 |
规范 |
默认值 |
可选 |
描述 |
---|---|---|---|---|---|
sample_size |
Integer | -1 , -2 , [1, |V|] |
-2 |
是 | 设为-1 时,采样log10(|V|) 个点(|V| 为图中点数量),或自定义一个在[1, |V|] 区间内的采样数;设为-2 时,不进行采样 |
max_path_length |
Integer | >0 | / | 是 | 只考虑长度小于或等于该值的最短路径;注意此参数不影响考虑的点对总数 |
return_id_uuid |
String | uuid , id , both |
uuid |
是 | 在结果中使用_uuid 、_id 或同时使用两者来表示点 |
limit |
Integer | ≥-1 | -1 |
是 | 限制返回的结果数;-1 返回所有结果 |
order |
String | asc , desc |
/ | 是 | 根据betweenness_centrality 对结果排序 |
文件回写
CALL algo.betweenness_centrality.write("hdc_betweenness", {
params: {
return_id_uuid: "id"
},
return_params: {
file: {
filename: "betweenness_centrality"
}
}
})
algo(betweenness_centrality).params({
projection: "hdc_betweenness",
return_id_uuid: "id"
}).write({
file: {
filename: "betweenness_centrality"
}
})
结果:
_id,betweenness_centrality
Mark,0.133333
Jay,0.0666667
Ann,0.133333
Sue,0
Dave,0.333333
Billy,0
May,0.0666667
数据库回写
将结果中的betweenness_centrality
值写入指定点属性。该属性类型为float
。
CALL algo.betweenness_centrality.write("hdc_betweenness", {
params: {},
return_params: {
db: {
property: 'bc'
}
}
})
algo(betweenness_centrality).params({
projection: "hdc_betweenness"
}).write({
db:{
property: 'bc'
}
})
完整返回
CALL algo.betweenness_centrality("hdc_betweenness", {
params: {
max_path_length: 2,
return_id_uuid: "id",
order: "desc",
limit: 3
},
return_params: {}
}) YIELD bc
RETURN bc
exec{
algo(betweenness_centrality).params({
max_path_length: 2,
return_id_uuid: "id",
order: "desc",
limit: 3
}) as bc
return bc
} on hdc_betweenness
结果:
_id | betweenness_centrality |
---|---|
Dave | 0.2 |
May | 0.066667 |
Mark | 0.066667 |
流式返回
CALL algo.betweenness_centrality("hdc_betweenness", {
params: {
return_id_uuid: "id"
},
return_params: {
stream: {}
}
}) YIELD r
FILTER r.betweenness_centrality = 0
RETURN count(r)
exec{
algo(betweenness_centrality).params({
return_id_uuid: "id"
}).stream() as r
where r.betweenness_centrality == 0
return count(r)
} on hdc_betweenness
结果:2
在分布式投影上运行算法
创建分布式投影
将图集全部投影到shard服务器上并命名为dist_betweenness
:
create().projection("dist_betweenness", {
nodes: {"*": ["*"]},
edges: {"*": ["*"]},
direction: "undirected",
load_id: true
})
参数
算法名:betweenness_centrality
参数名 |
类型 |
规范 |
默认值 |
可选 |
描述 |
---|---|---|---|---|---|
sample_rate |
Float | (0, 1] | 1 | 是 | 采样一定比例的边进行计算 |
limit |
Integer | ≥-1 | -1 |
是 | 限制返回的结果数;-1 返回所有结果 |
order |
String | asc , desc |
/ | 是 | 根据betweenness_centrality 分值对结果排序 |
文件回写
CALL algo.betweenness_centrality.write("dist_betweenness", {
params: {},
return_params: {
file: {
filename: "betweenness_centrality"
}
}
})
algo(betweenness_centrality).params({
projection: "dist_betweenness"
}).write({
file: {
filename: "betweenness_centrality"
}
})
结果:
_id,betweenness_centrality
Mark,0.133333
Jay,0.0666667
Ann,0.133333
Sue,0
Dave,0.333333
Billy,0
May,0.0666667
数据库回写
将结果中的betweenness_centrality
值写入指定点属性。该属性类型为double
。
CALL algo.betweenness_centrality.write("dist_betweenness", {
params: {},
return_params: {
db: {
property: 'bc'
}
}
})
algo(betweenness_centrality).params({
projection: "dist_betweenness"
}).write({
db:{
property: 'bc'
}
})