概述
p-Cohesion算法能识别网络中彼此高度关联的一组参与者(节点),并由内聚子图(Cohesive Subgraph)表示。通过了解各组内的连通性和相互依赖程度,该算法能够帮助深入分析图结构及其影响。
p-Cohesion的概念最初是由S. Morris在大群人相互作用的传染模型中提出的:
- S. Morris, Contagion. The Review of Economic Studies, 67(1), 57–78 (2000)
基本概念
p-Cohesion
关于群体的Cohesion(凝聚力),一个很自然的衡量标准是:与非成员相比,群体内成员之间互相联系的相对概率。令常数p∈(0,1),p-Cohesion是一个连通子图,其中每个节点至少有占比为p的邻居在该子图中,每个节点在子图外的邻居占比不超过(1−p)。
与其他内聚子图模型相比,p-Cohesion模型具有两个明显的优势:
- p值较大时,p-Cohesion能同时保证内部的凝聚性和外部的稀疏性。
- 在很多场景下,由于图中各节点度的差异,考虑邻居的占比相较于考虑固定的邻居数(比如 k-Core)更为合适。
在下面的示例图中,假设p=0.6,每个节点旁的灰色标签表示该节点在p-cohesion中所需的最小邻居数。
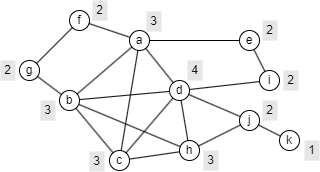
以下分别是包含节点a和节点j的最小(指节点数量)p-cohesion 子图。
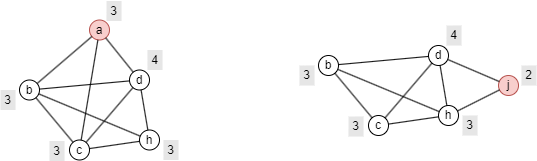
嬴图的p-Cohesion算法寻找包含查询节点的近似最小p-cohesion子图,并以节点集的形式返回子图。
特殊说明
- p-Cohesion算法忽略边的方向,按照无向边进行计算。
语法
- 命令:
algo(p_cohesion)
- 参数:
名称 |
类型 |
规范 |
默认 |
可选 |
描述 |
---|---|---|---|---|---|
ids / uuids | []_id / []_uuid |
/ | / | 是 | 待查询节点的ID/UUID;分别寻找包含每个查询节点的近似最小p-cohesion子图;忽略则查询所有节点 |
p | float | (0,1) | / | 否 | p-Cohesion子图中每个节点至少有占比为p的邻居在该子图中,在子图外的邻居数占比不超过(1 − p) |
示例
示例图如下:
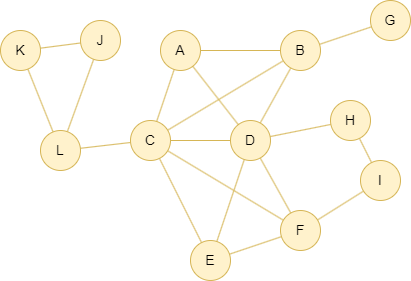
文件回写
配置项 |
回写内容 |
描述 |
---|---|---|
filename | subgraphN : _id ,_id ,... |
每个p-cohesion子图中的节点 |
algo(p_cohesion).params({
ids: ['A', 'I'],
p: 0.7
}).write({
file: {
filename: "cohesion"
}
})
统计值:num of subgraphs = 2
结果:文件cohesion
subgraph0:D,C,B,F,A,E,
subgraph1:D,C,F,B,H,E,A,I,